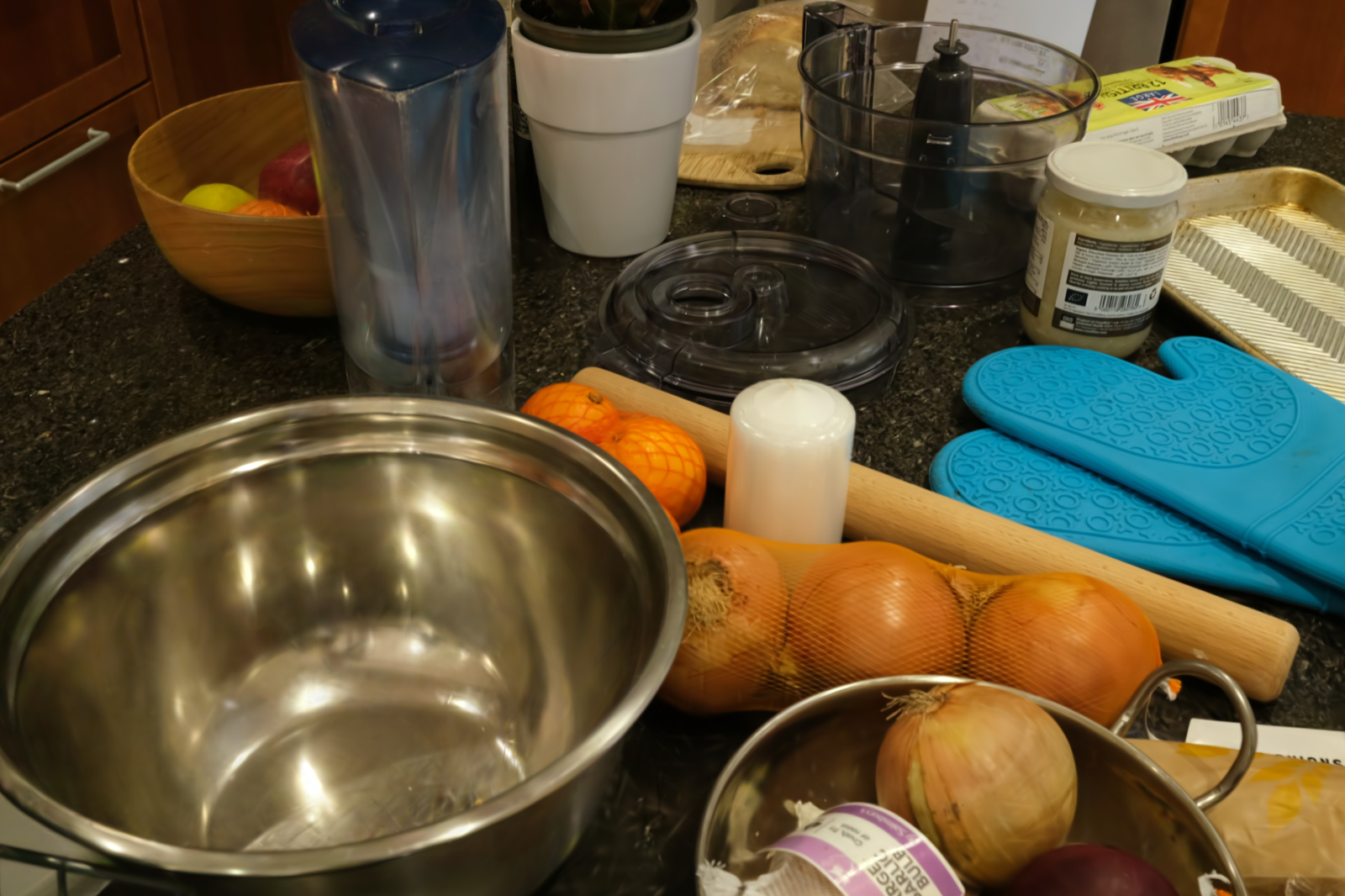
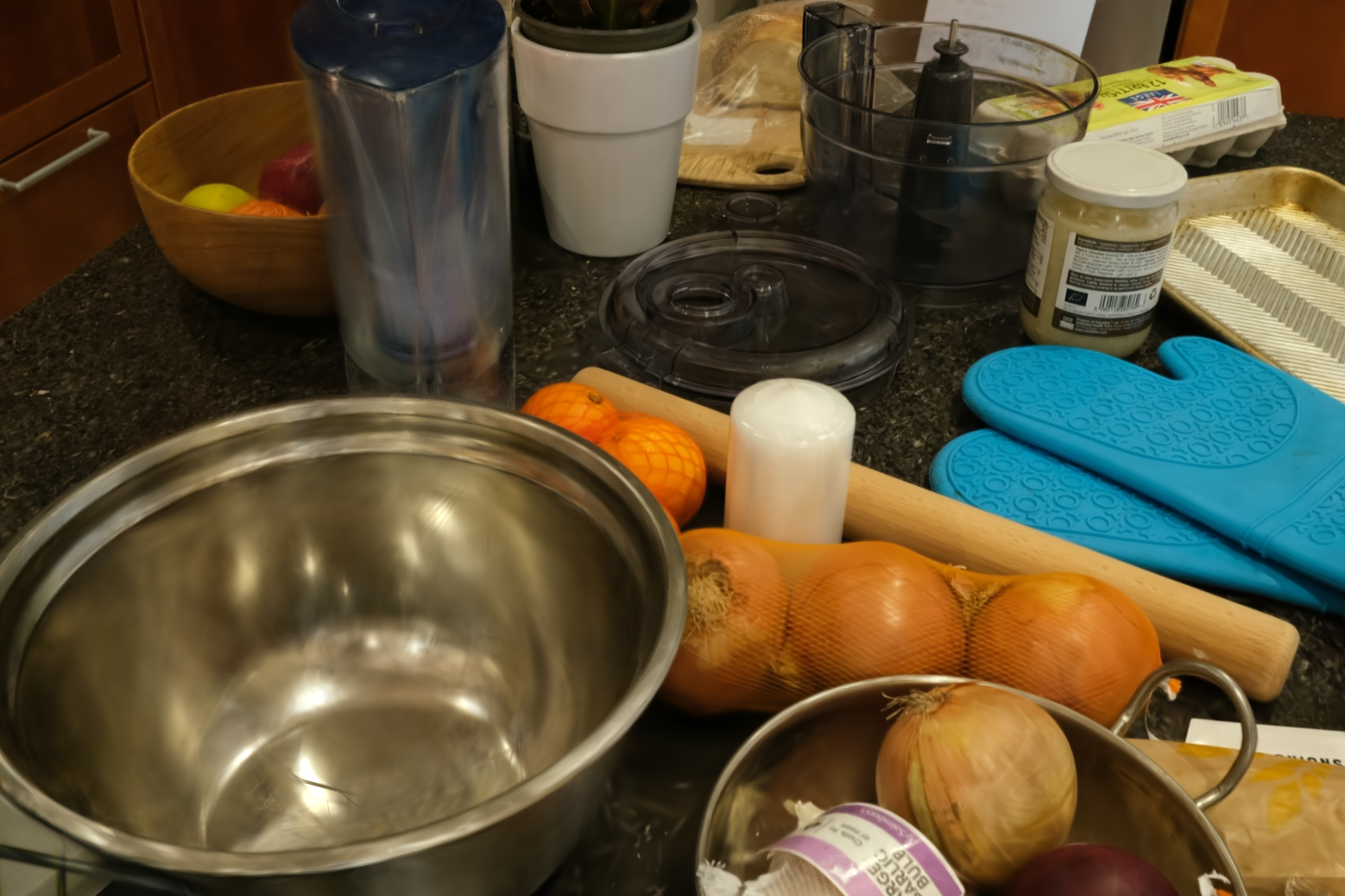
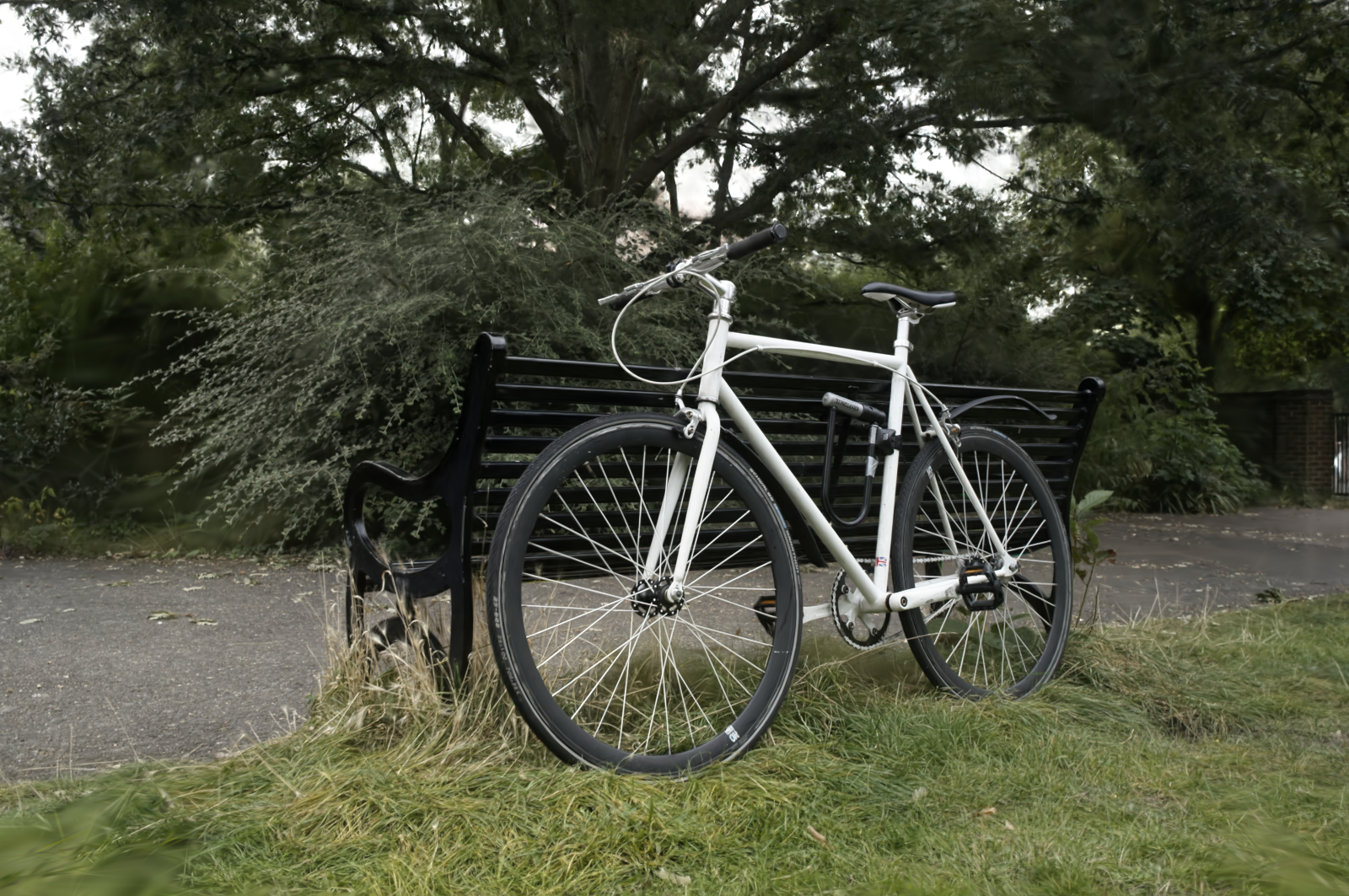
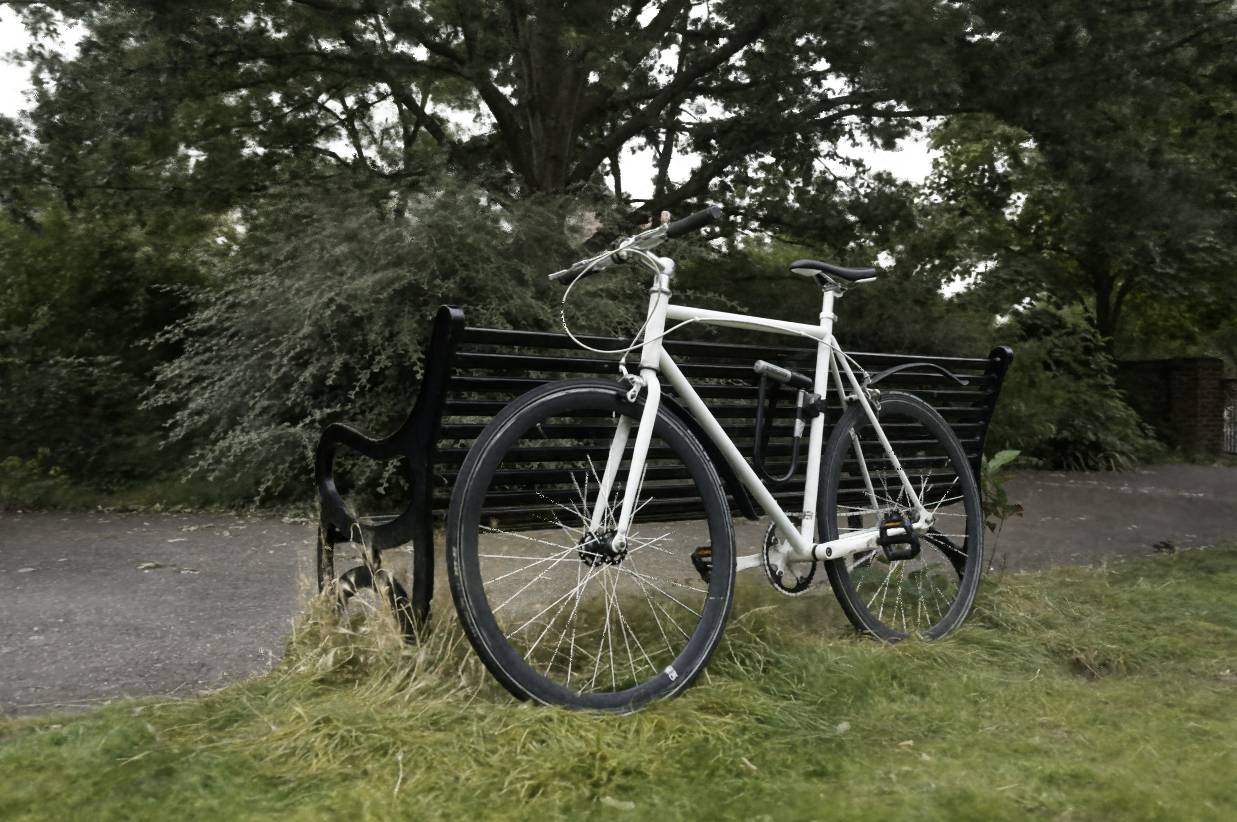
To assess NieR's ability to render scenes with realistic lighting and fine details, we compare it against Gaussian Splatting as the baseline method. We employ established quantitative metrics, including Peak Signal-to-Noise Ratio (PSNR), Learned Perceptual Image Patch Similarity (LPIPS), and Structural Similarity Index (SSIM). These metrics evaluate the similarity between rendered images and ground truth data, quantifying visual fidelity and detail preservation. we compare our method with Gaussian Splatting, Mip-NeRF360, InstantNGP, and Plenoxels on seven scenes from the Mip-Nerf360 dataset and two scenes from the Tanks and Temples dataset [2017]. For the full evaluation please check the paper and the supplemental.